Motivation
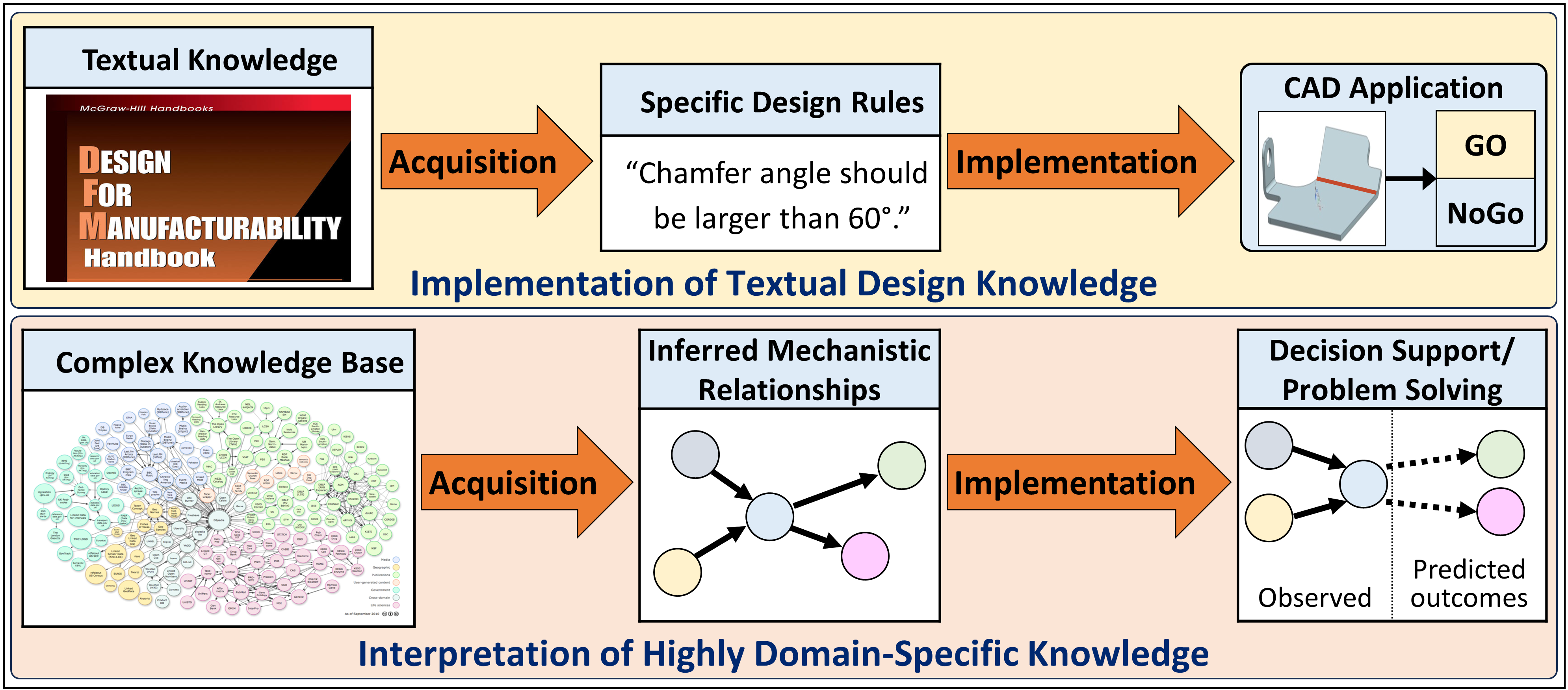
Insightful human knowledge on design and manufacturing has been maintained in various forms: plain text, unorganized data, or complex knowledge bases. However, such knowledge may not be readily understandable by computer.
Therefore, people spend a considerable amount of time acquiring and implementing meaningful knowledge for design & manufacturing.
Challenges
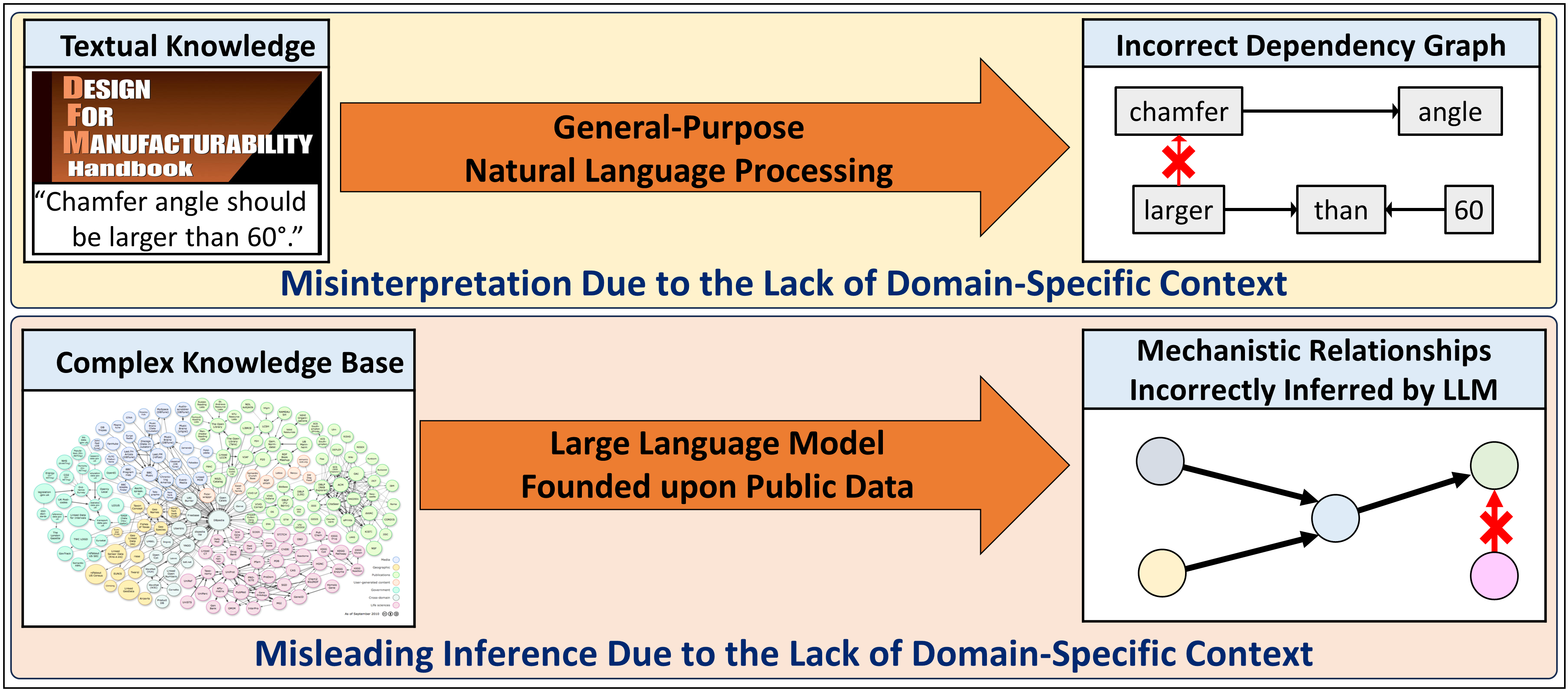
To improve the efficiency of knowledge acquisition and implementation, natural language processing and other machine learning methods have been studied.
However, since it is difficult to access enough data for design and manufacturing, general-purpose tools/models trained from public data might extract misleading knowledge, due to the lack of domain-specific context.
Approach
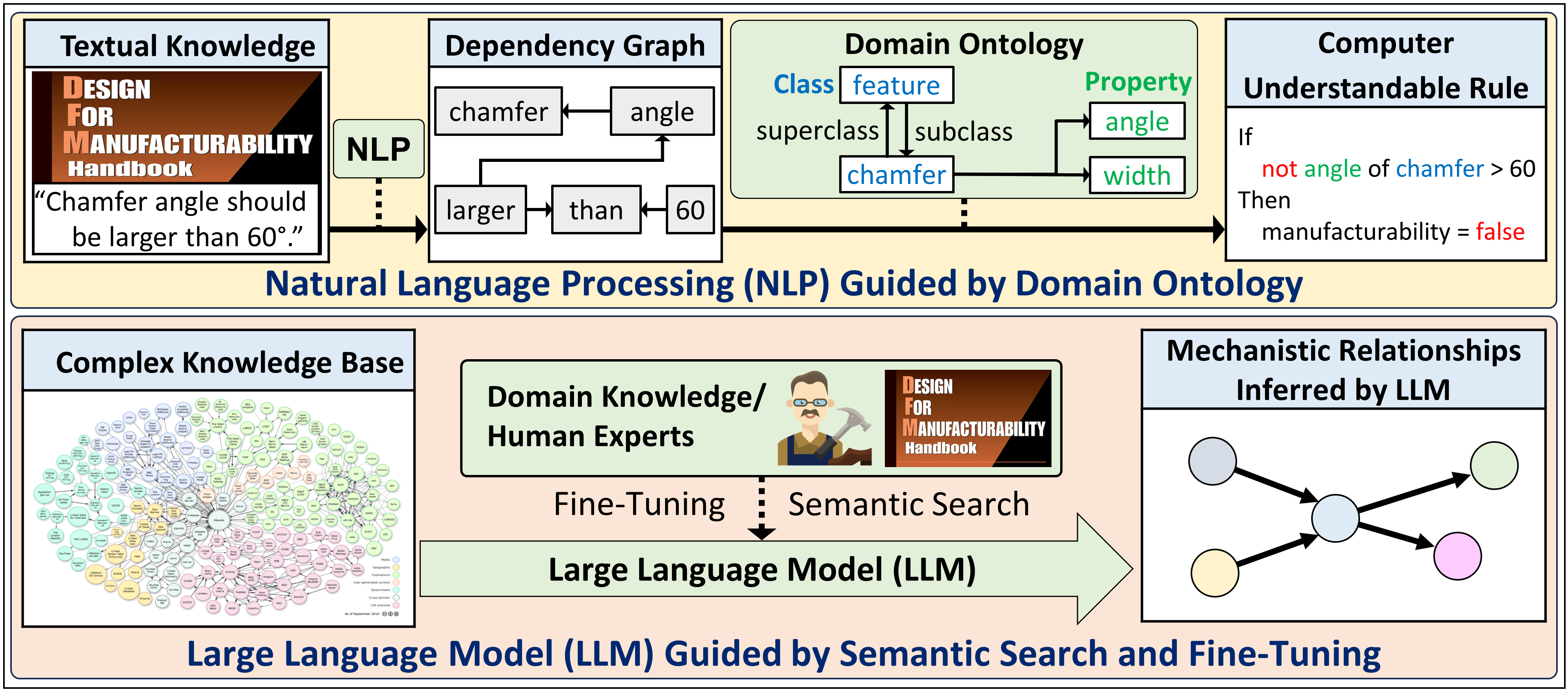
In this context, this research aims to extract computer-understandable domain-specific knowledge, especially from plain text and complex knowledge bases.
Here, domain-specific knowledge is incorporated in a computer-understandable way (e.g., ontology, semantic search) or large language models are fine-tuned with a minimal amount of domain-specific data.
Value
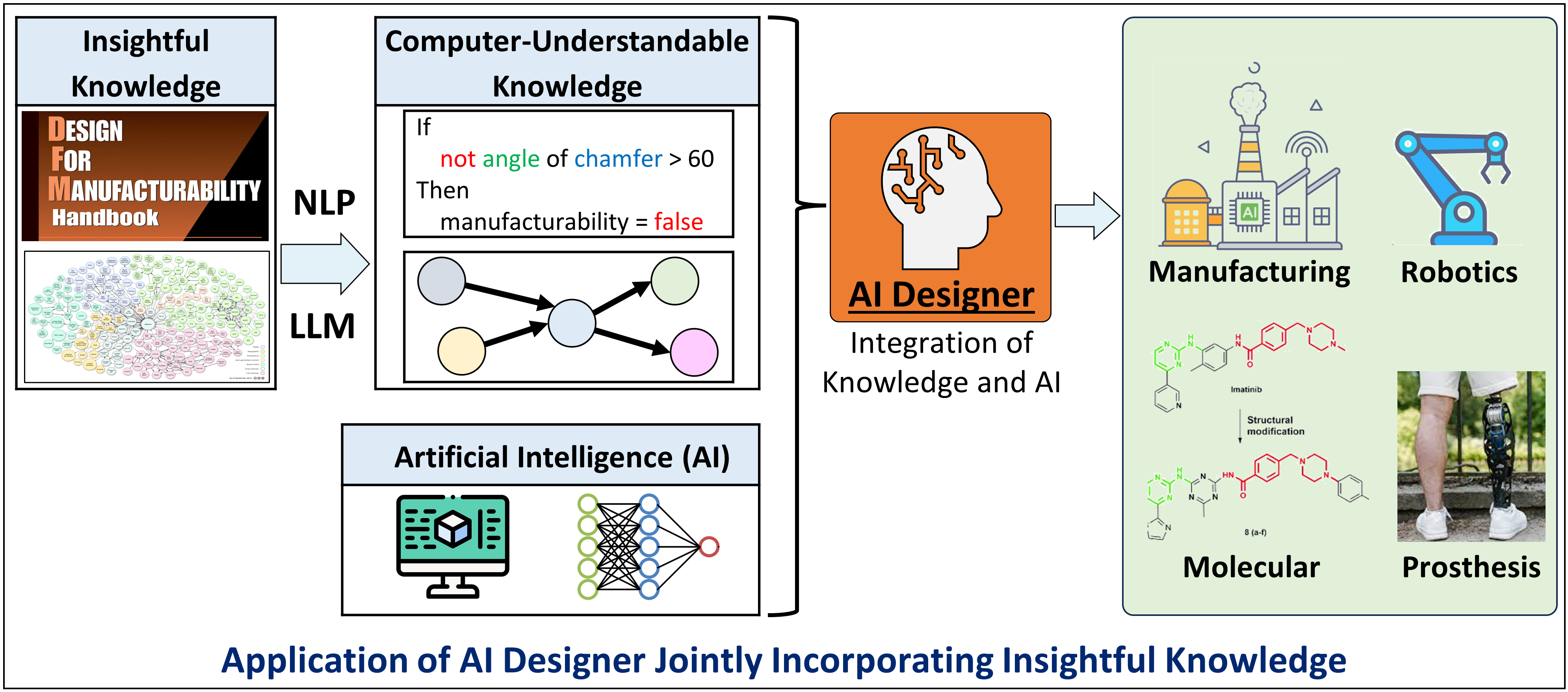
This work will pave the way to extract and implement computer-understandable domain knowledge in any area, such that insightful knowledge can be readily integrated with state-of-the-art artificial intelligence.
Not only for manufacturing, this approach can be generalizable to high-value applications that rely on experts' knowledge, such as medical and material design.