Motivation
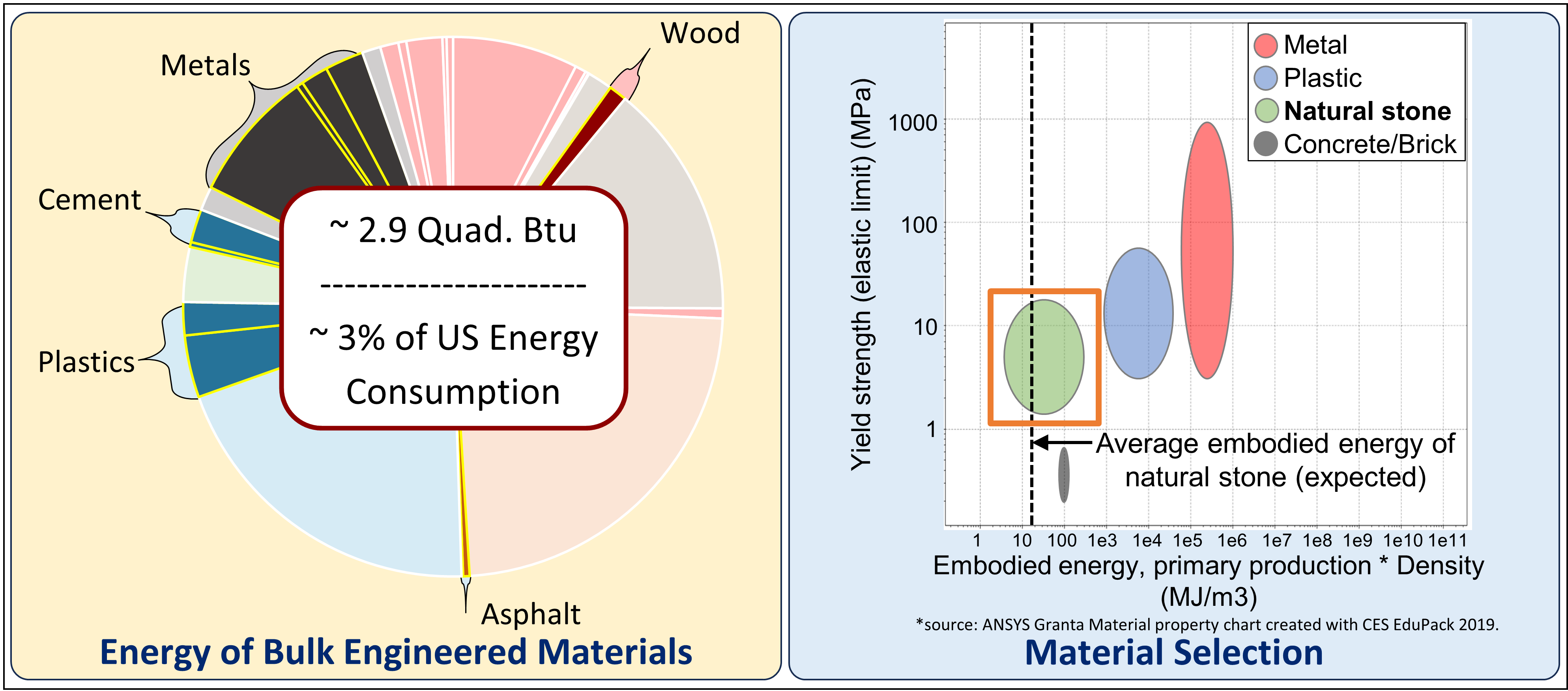
The operations of buildings account for up to 30% of global energy consumption and energy-related emissions, where up to 25% of its energy is attributed to the embodied energy of construction materials.
In this context, the use of locally sourced natural materials, such as natural stone, is regarded as a sustainable alternative in the construction industry.
Challenges
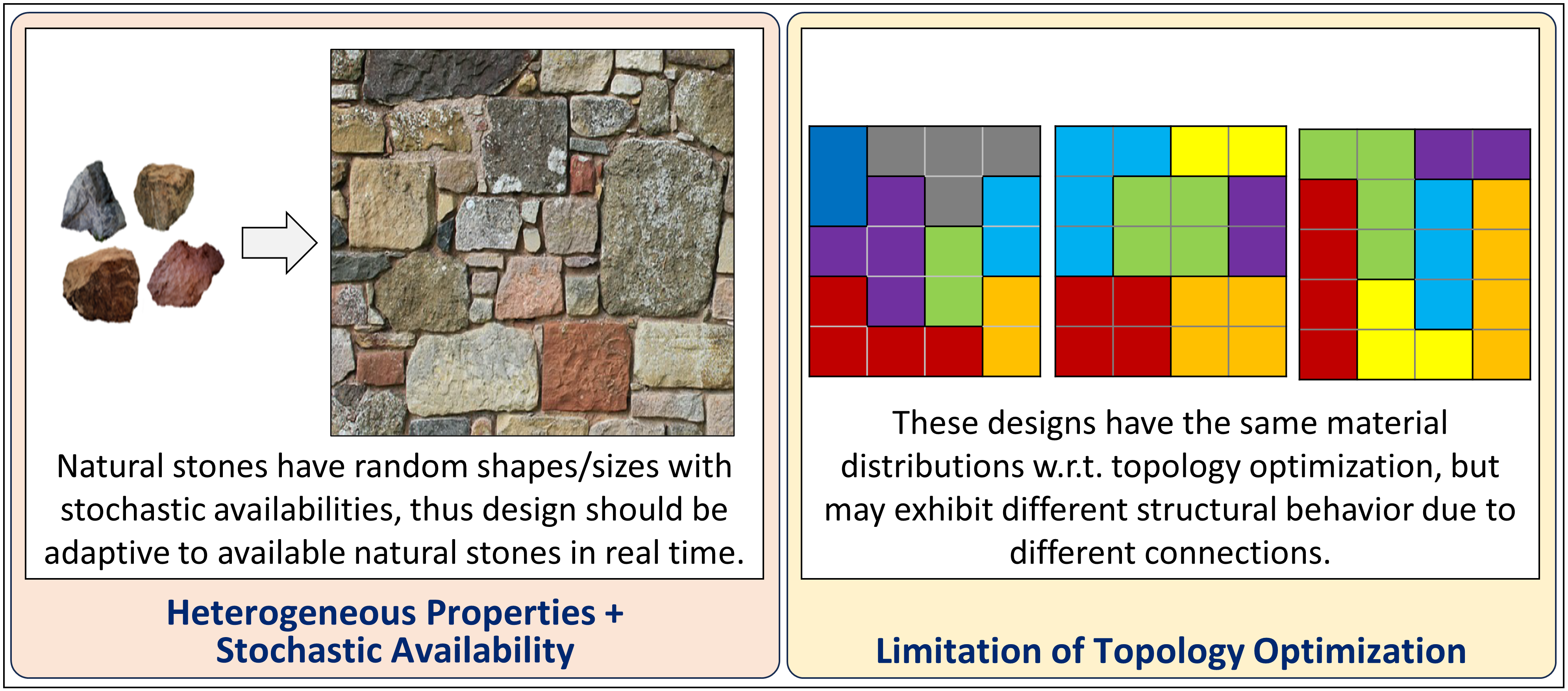
However, stone masonry design is challenging to solve with topology optimization methods, since it involves the sequential organization of natural stones for proper load transfer, where the availability/shape/size of natural stones are random.
Therefore, stone masonry design requires adaptive utilization of random stones to achieve the desirable structural behavior, similar to what highly experienced experts are doing.
Approach
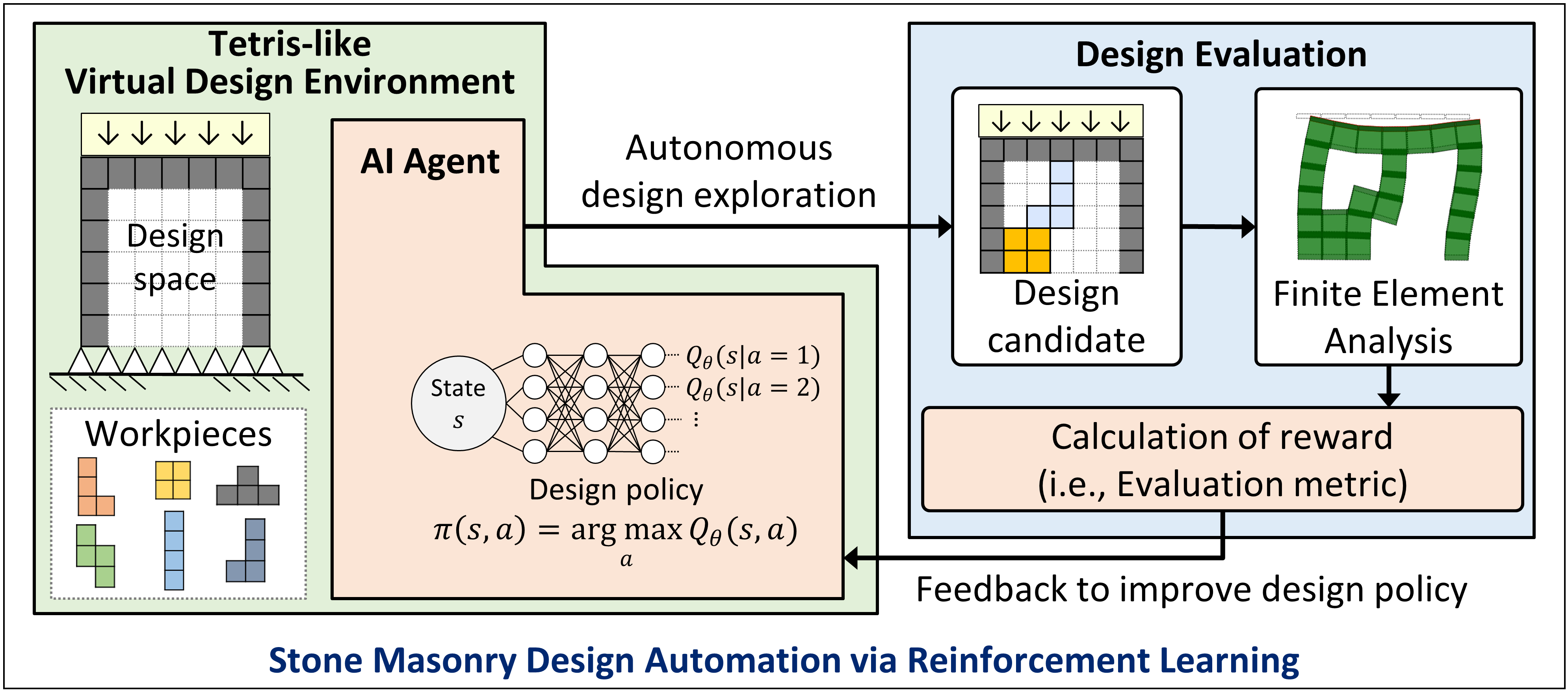
Inspired by the similarity between Tetris and stone masonry, a virtual design environment called MasonTris is developed, where AI can learn stone masonry design via reinforcement learning.
Inside MasonTris, AI autonomously explore different design candidates by playing Tetris-like games, and then the design candidates are evaluated with finite element analysis. The performance of each design candidate (i.e., reward) is fed back to the AI to continuously improve its design policy from experience.
Value
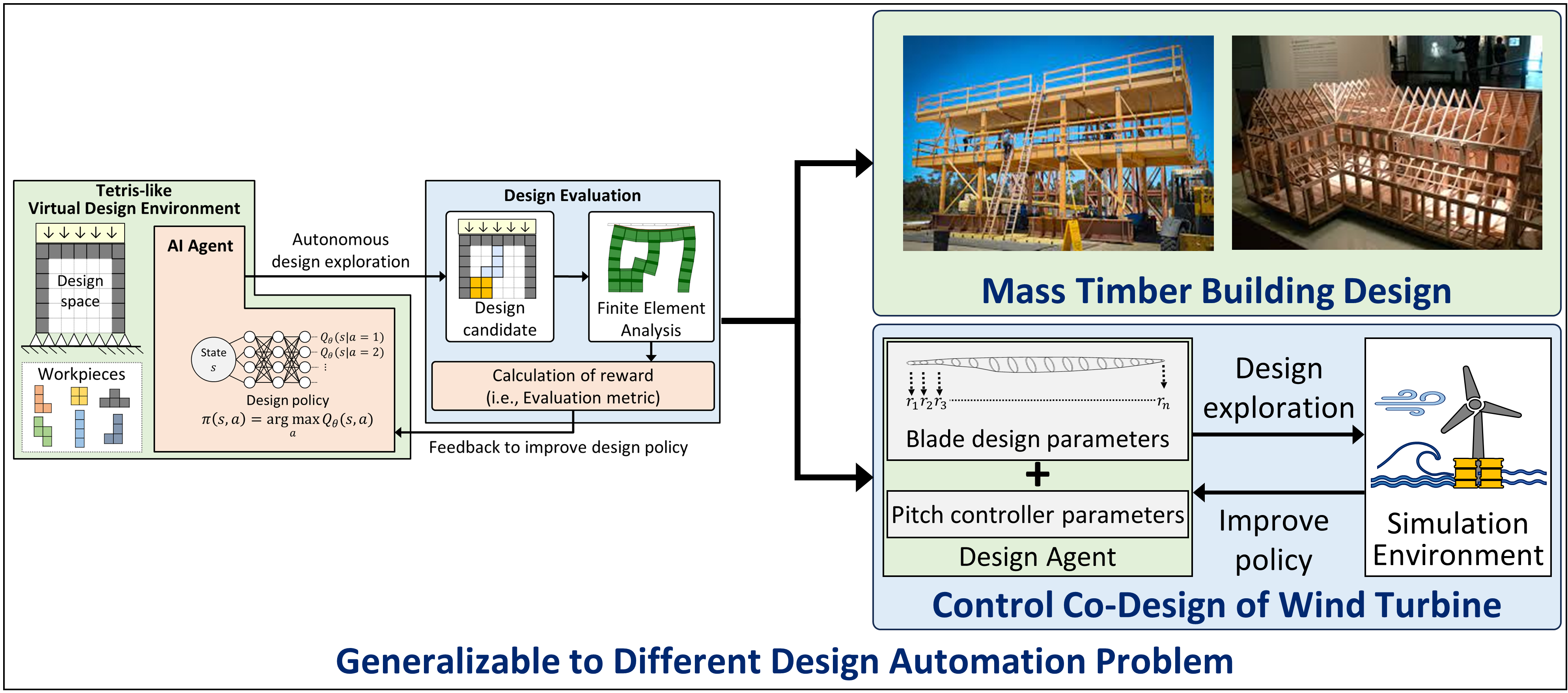
This work demonstrates the use of reinforcement learning for autonomous learning of an effective design policy, which is adaptable to the materials with heterogeneous properties and/or stochastic availability.
Therefore, this approach can be generalizable to different types of sustainable materials, such as locally sourced natural materials (e.g., timber) and recycled materials with different usage history (e.g., reclaimed plastic).